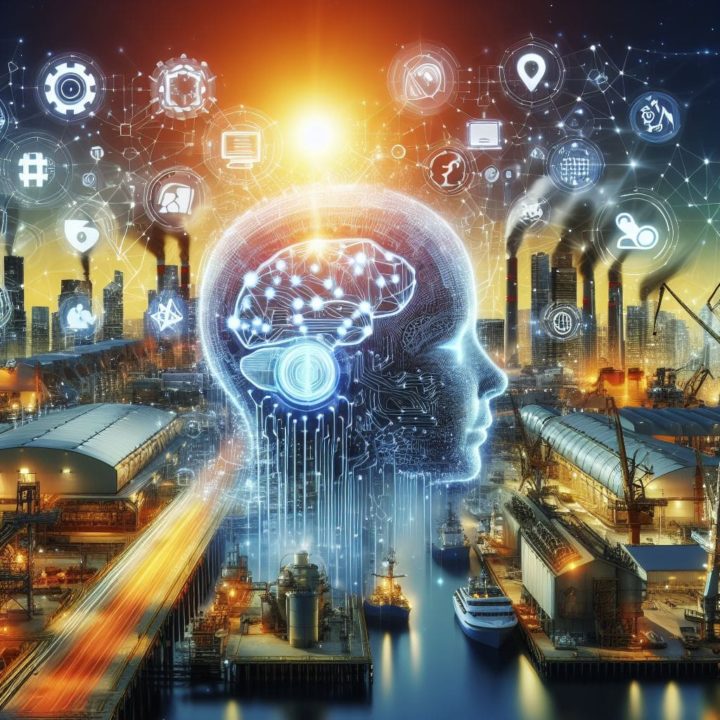
In today’s digital era, the amount of data generated and collected is growing at an unimaginable pace. With the advent of technology, every aspect of our lives has become interconnected, resulting in an overwhelming abundance of information. Consequently, big data has emerged as a critical asset that holds immense potential for businesses, organizations, and individuals alike.
However, the real challenge lies in harnessing this data to gain valuable insights and make informed decisions. Traditional methods of data analysis often fall short when it comes to handling the massive volume, velocity, and variety of big data. That’s where the power of artificial intelligence (AI) comes into play.
AI, with its ability to replicate human intelligence and learn from experience, is revolutionizing the field of big data analytics. By employing sophisticated algorithms and machine learning techniques, AI platforms can process, analyze, and interpret vast amounts of data in real-time, enabling organizations to extract invaluable insights and uncover hidden patterns and trends.
A New Era: Artificial Intelligence and Big Data Analytics
The rapid advancements in technology have brought about a new era in the field of data analytics. With the ever-increasing amounts of data being generated, traditional methods of analysis are no longer sufficient to extract meaningful insights. This is where artificial intelligence (AI) comes into play.
AI is revolutionizing big data analytics by providing the tools and techniques to process, analyze, and interpret large volumes of data with incredible speed and accuracy. With AI, businesses can now uncover hidden patterns, correlations, and trends that were previously impossible to identify.
One of the key benefits of using AI in big data analytics is its ability to automate the entire process. AI algorithms can handle complex tasks such as data cleansing, data integration, and data visualization, freeing up analysts to focus on more strategic activities.
In addition, AI can also enhance predictive modeling in big data analytics. By leveraging machine learning algorithms, AI can analyze historical data to identify patterns and make accurate predictions about future outcomes. This allows businesses to make data-driven decisions and gain a competitive edge.
Furthermore, AI can provide real-time insights into big data analytics. With the ability to process and analyze data as it is generated, AI can identify anomalies, detect fraud, and highlight important trends in real-time. This empowers businesses to respond quickly to changing market conditions and make timely decisions.
In conclusion, the combination of artificial intelligence and big data analytics marks a new era in the field of data analysis. With AI, businesses can unlock the true potential of their big data, gaining valuable insights and driving innovation. As AI continues to evolve, the possibilities for leveraging big data analytics are endless.
The Role of Artificial Intelligence in Big Data Analytics
Artificial intelligence (AI) has become an integral part of big data analytics. With the increasing amount of data being generated every day, AI is essential for organizations to make sense of this vast amount of information.
AI enables data analytics to go beyond traditional approaches by empowering machines to learn from and analyze large datasets. This allows organizations to uncover patterns, trends, and insights that would otherwise be impossible to discover manually.
One key role of AI in big data analytics is its ability to process and analyze data at a much faster rate than humans. AI algorithms can quickly sort through massive amounts of data, identify correlations, and derive meaningful insights in real-time.
Furthermore, AI can automate repetitive tasks in data analytics, freeing up human analysts to focus on more complex and strategic analysis. For example, AI can automate data cleansing, data transformation, and data visualization, allowing analysts to spend more time on interpreting results and making informed decisions.
AI also enhances the accuracy and precision of big data analytics. By using sophisticated algorithms, AI systems can detect anomalies, outliers, and inconsistencies in data that humans might overlook. This improves the quality of insights and recommendations derived from analytics, leading to better business outcomes.
Another important role of AI in big data analytics is its ability to provide personalized recommendations and predictions. By understanding individual behaviors and preferences, AI algorithms can generate tailored recommendations for products, services, and content. This improves customer satisfaction and drives business growth.
- In conclusion, artificial intelligence plays a crucial role in big data analytics by enabling organizations to effectively analyze and extract value from large datasets. With its speed, automation, accuracy, and personalization capabilities, AI transforms data analytics into a more efficient and powerful process.
Machine Learning Algorithms for Big Data Analysis
In the era of big data, the amount of data generated and collected is increasing exponentially. With this exponential growth, the need for efficient and accurate analysis of the data has become paramount. Machine learning algorithms have emerged as a powerful tool for data analysis, as they can automatically learn patterns and relationships in the data, without the need for explicit programming.
Machine learning algorithms are designed to process large volumes of data, making them well-suited for big data analysis. These algorithms use computational intelligence to extract useful insights and information from the vast amount of data available. With the advent of artificial intelligence, machine learning algorithms have become even more sophisticated and powerful.
There are various machine learning algorithms that are commonly used for big data analysis. Some of the popular algorithms include:
- Decision Trees: Decision trees are tree-like models that use a sequence of decisions to classify or predict outcomes.
- Random Forests: Random forests are an ensemble technique that combines multiple decision trees to make more accurate predictions.
- Support Vector Machines: Support vector machines are binary classifiers that find the hyperplane that best separates data points of different classes.
- Naive Bayes: Naive Bayes is a probabilistic classifier that predicts the probability of a data point belonging to a certain class.
- Neural Networks: Neural networks are a powerful class of machine learning algorithms inspired by the structure and function of the human brain.
These machine learning algorithms can handle enormous amounts of data and can extract valuable insights and patterns that can be used for various purposes, such as predictive analytics, anomaly detection, and recommendation systems. They can also be used to automate tasks and make data-driven decisions.
In conclusion, machine learning algorithms play a crucial role in big data analysis. With their ability to process and analyze large volumes of data, combined with artificial intelligence, these algorithms have revolutionized the way we analyze and extract value from data. As big data continues to grow, machine learning algorithms will continue to evolve and become even more powerful, enabling us to derive even deeper insights from the massive amounts of data at our disposal.
Deep Learning Techniques for Big Data Processing
As the volume of data continues to grow exponentially, it becomes increasingly challenging to extract meaningful insights and make informed decisions. This is where big data analytics comes into play, helping organizations transform vast amounts of data into valuable knowledge. However, traditional analytics approaches are often unable to keep up with the scale and complexity of big data. This is where artificial intelligence (AI) and deep learning techniques come in.
Deep learning is a subset of machine learning that involves training artificial neural networks with multiple layers to learn from vast amounts of data. It mimics the way the human brain processes information, analyzing patterns and making predictions. When it comes to big data processing, deep learning techniques have proven to be highly effective in handling the immense volume, velocity, and variety of data.
1. Neural Networks
At the core of deep learning techniques are neural networks, which consist of interconnected nodes or “neurons.” Each node takes input and applies a mathematical function to it, ultimately producing an output. By stacking multiple layers of neurons, neural networks can learn complex patterns and relationships within the data.
Neural networks have revolutionized big data processing, enabling the development of models that can process and analyze vast amounts of data with speed and accuracy. They can handle structured, unstructured, and semi-structured data, making them ideal for big data analytics tasks.
2. Convolutional Neural Networks
Convolutional Neural Networks (CNNs) are a type of neural network specifically designed for image recognition and processing. They are particularly effective in analyzing large image datasets, extracting features, and making accurate predictions.
CNNs have been instrumental in various fields, such as computer vision, medical image analysis, and autonomous vehicles. By combining deep learning with big data analytics, CNNs can process massive image datasets and deliver valuable insights for decision-making.
In conclusion, deep learning techniques offer a powerful solution for big data processing. With their ability to handle the immense volume and complexity of data, deep learning algorithms, such as neural networks and convolutional neural networks, enable organizations to extract meaningful insights and make informed decisions in the era of big data and artificial intelligence.
Natural Language Processing for Big Data Text Analytics
In the era of big data, the ability to extract meaningful insights from unstructured text data has become critical. Natural Language Processing (NLP) is a branch of artificial intelligence (AI) that focuses on the interaction between human language and computers. NLP techniques are revolutionizing big data analytics, allowing organizations to gain valuable knowledge from vast amounts of textual information.
With the exponential growth of data, traditional methods of text analysis are no longer sufficient. NLP enables computers to understand, interpret, and derive meaning from human language in a way that was previously only possible for humans. By applying NLP algorithms to big data, organizations can transform unstructured text into structured and actionable insights.
One of the key challenges in big data text analytics is the vast amount of noise in unstructured text data. NLP techniques can help filter out irrelevant information and focus on the most relevant content. By identifying patterns in the data, NLP can provide valuable insights for decision-making and strategy development.
NLP can also be used to extract entities, such as people, organizations, and locations, from text data. This enables organizations to perform deeper analysis by identifying key players and their relationships. Furthermore, sentiment analysis can be performed using NLP techniques to understand the emotions and opinions expressed in large volumes of text.
With the help of NLP, organizations can uncover hidden patterns, trends, and relationships in big data text analytics. This allows them to make data-driven decisions, detect potential risks, and capitalize on new opportunities. The combination of artificial intelligence and big data analytics is creating a powerful synergy that is revolutionizing the way organizations gain insights from textual data.
In conclusion, natural language processing is playing a crucial role in revolutionizing big data text analytics. By leveraging NLP techniques, organizations can overcome the challenges of analyzing unstructured text data and extract valuable insights. As AI continues to advance, the capabilities of NLP are expected to further transform the field of big data analytics.
Image Recognition and Computer Vision for Big Data
With the advancements in artificial intelligence, big data analytics has been revolutionized with the use of image recognition and computer vision technologies. These technologies have enabled machines to understand and interpret visual data, leading to a wide range of applications in various industries.
Image recognition algorithms allow computers to analyze, identify, and understand images or patterns within images. This technology is used in a variety of fields, including healthcare, manufacturing, retail, and security. For example, in healthcare, image recognition can be used to detect diseases or anomalies in medical images, aiding in the early diagnosis and treatment of patients.
Computer vision, on the other hand, provides computers with the ability to interpret and understand the visual world. This allows machines to analyze and extract information from images or videos, enabling a range of applications such as object recognition, image classification, and video surveillance. The data generated from computer vision can provide valuable insights for businesses, helping them make data-driven decisions.
By combining image recognition and computer vision with big data analytics, organizations can unlock the true potential of their data. They can process and analyze large volumes of visual data, extracting valuable information and patterns that were previously difficult to identify. This can lead to improved decision-making, increased operational efficiency, and enhanced customer experiences.
Furthermore, the integration of artificial intelligence with big data analytics allows for real-time image analysis and interpretation. This enables businesses to detect and respond to events or patterns as they occur, leading to faster and more accurate decision-making.
In conclusion, the use of image recognition and computer vision technologies in big data analytics has revolutionized the way organizations analyze and interpret visual data. With the advancements in artificial intelligence, businesses can now unlock the valuable insights hidden within large volumes of image and video data, leading to improved decision-making and increased operational efficiency.
Predictive Analytics using Artificial Intelligence
Predictive analytics is a powerful tool that leverages the power of artificial intelligence (AI) to make accurate predictions based on historical data. This technology can transform the way businesses operate by providing insights into future trends, customer behavior, and market dynamics.
With the advent of AI, predictive analytics has become more intelligent and efficient. AI algorithms can analyze large volumes of data in real-time, identifying patterns and correlations that humans may not be able to detect. This enables businesses to make data-driven decisions and take proactive measures to optimize their operations.
One of the key advantages of using AI in predictive analytics is its ability to handle complex and unstructured data. Traditional analytics methods often struggle with messy, unorganized data sets, but AI can process and analyze this data with ease. This means that businesses can extract valuable insights from a wide variety of sources, including social media data, sensor data, and text data.
Benefits of Predictive Analytics using Artificial Intelligence:
1. Improved Accuracy: AI algorithms can analyze large volumes of data with precision, resulting in more accurate predictions and insights.
2. Time and Cost Savings: By automating the data analysis process, AI can save businesses time and money compared to manual methods.
3. Proactive Decision Making: Predictive analytics allows businesses to anticipate future trends and take proactive measures to stay ahead of the competition.
4. Personalized Experiences: By analyzing customer data, AI-powered predictive analytics can provide personalized recommendations and experiences, enhancing customer satisfaction and loyalty.
In conclusion, incorporating artificial intelligence into predictive analytics can revolutionize the way businesses analyze and utilize data. With its ability to process large volumes of complex data, AI enables businesses to make accurate predictions, optimize operations, and provide personalized experiences to customers. As AI continues to evolve, the potential for predictive analytics to drive innovation and business growth is limitless.
Sentiment Analysis for Big Data Insights
Sentiment analysis is a powerful tool in artificial intelligence that revolutionizes big data analytics. It allows organizations to gain valuable insights from large amounts of data by analyzing the sentiment expressed in text and other forms of unstructured data. With the advent of machine learning and natural language processing algorithms, sentiment analysis has become more accurate and efficient than ever before.
By applying sentiment analysis to big data, organizations can uncover patterns and trends in customer feedback, social media posts, online reviews, and more. They can identify positive and negative sentiments associated with their products or services, helping them understand customer satisfaction and make data-driven decisions to improve their offerings.
One of the challenges of sentiment analysis for big data is the sheer volume of data that needs to be processed. With the vast amount of information available, manual analysis is not feasible. This is where artificial intelligence comes in. Machine learning algorithms can analyze large datasets in real-time, allowing organizations to gain insights and take immediate action.
Another challenge is the diversity of data sources. Sentiment analysis algorithms must be able to handle different types of data, including text, images, and videos. This requires advanced techniques such as deep learning, which enables the analysis of complex data structures.
In conclusion, sentiment analysis is a crucial component of big data analytics. It enables organizations to extract valuable insights from vast amounts of data, helping them make informed decisions and improve their products and services. With the advancements in artificial intelligence and big data technology, sentiment analysis is becoming more accurate and efficient, opening up new possibilities for data-driven businesses.
Speech Recognition for Big Data Voice Analytics
In the era of big data, speech recognition technology is revolutionizing the way we extract valuable insights from spoken word data. With the advent of artificial intelligence, the capabilities of speech recognition algorithms have reached new heights, making it an essential tool for analyzing voice data in the context of big data analytics.
Big data encompasses a vast amount of information, including text, images, videos, and most importantly, voice data. Voice data, in the form of audio recordings or transcriptions, contains valuable insights that can unlock hidden patterns, sentiments, and even intent. However, manually analyzing this data is time-consuming, resource-intensive, and prone to human error.
Artificial intelligence-powered speech recognition systems excel at converting voice data into actionable information. By leveraging machine learning algorithms, these systems can transcribe spoken words with high accuracy and speed. This allows organizations to process large volumes of voice data quickly and cost-effectively.
Speech recognition technology is not only limited to transcribing voice data; it can also perform various analysis tasks. For instance, sentiment analysis can be applied to determine the emotional tone of a spoken conversation, enabling organizations to gauge customer satisfaction or identify potential issues.
Furthermore, speech recognition algorithms can identify patterns and trends within voice data, providing valuable insights into customer behavior, preferences, and needs. This can assist organizations in making data-driven decisions, improving customer experiences, and optimizing business processes.
Another important use case for speech recognition in big data analytics is voice biometrics. By analyzing unique vocal characteristics, such as pitch, tone, and timbre, these systems can authenticate individuals based on their voice. Voice biometrics can enhance security measures, streamline authentication processes, and enable more personalized user experiences.
In conclusion, speech recognition technology powered by artificial intelligence is transforming big data voice analytics. It offers unmatched capabilities for processing and analyzing voice data, unlocking valuable insights, and powering data-driven decision-making. With the ever-growing volume of voice data, organizations that leverage speech recognition technology are poised to gain a competitive edge in today’s data-driven world.
Optimization Algorithms for Enhanced Big Data Analytics
Artificial intelligence revolutionizes big data analytics by providing advanced algorithms and techniques to optimize the processing and analysis of vast amounts of data. Big data refers to the large volumes of structured and unstructured data that organizations collect and analyze to gain insights and make informed decisions.
Integrating intelligence into big data analytics enhances the accuracy and efficiency of data processing. Optimization algorithms play a crucial role in this process, as they help optimize various aspects of big data analytics, such as data storage, retrieval, and analysis.
These algorithms are designed to handle and process vast amounts of data effectively and efficiently. They aim to reduce the computational complexity and processing time required for analyzing big data. By using optimization algorithms, organizations can extract valuable insights from their big data faster and make data-driven decisions in real time.
Benefits of Optimization Algorithms in Big Data Analytics |
---|
1. Improved data processing speed: Optimization algorithms optimize the data processing workflow, enabling faster analysis of large datasets. |
2. Enhanced accuracy: These algorithms improve the accuracy of data analysis by minimizing errors and eliminating biases in the results. |
3. Resource optimization: Optimization algorithms help organizations optimize the allocation of resources, such as computing power and storage, to handle big data more efficiently. |
4. Scalability: These algorithms are designed to scale with the size and complexity of big data, ensuring the efficient processing of data as it continues to grow. |
5. Real-time analysis: Optimization algorithms enable real-time data analysis, allowing organizations to make timely and data-driven decisions. |
In conclusion, optimization algorithms play a crucial role in enhancing big data analytics by improving data processing speed, accuracy, resource allocation, scalability, and enabling real-time analysis. By leveraging artificial intelligence and these advanced algorithms, organizations can unlock the full potential of their big data and gain valuable insights to drive business growth and success.
Data Visualization with Artificial Intelligence
With the advent of big data and advancements in artificial intelligence (AI), data visualization has become an essential tool for analyzing and presenting complex data sets. By leveraging AI, data scientists and analysts can now extract valuable insights from vast amounts of data and communicate them through intuitive and visually appealing visualizations.
Artificial intelligence algorithms can process and analyze large data sets quickly and efficiently, enabling organizations to visualize complex patterns and trends in real-time. These algorithms can identify correlations and outliers, making it easier for analysts to interpret and communicate their findings. Furthermore, AI-powered visualization tools can automatically generate charts, graphs, and interactive dashboards, eliminating the need for manual data entry and formatting.
Improved Decision-Making
Data visualization coupled with AI allows businesses to make data-driven decisions more effectively. By transforming complex data into visual representations, decision-makers can easily grasp the information they need to take action. Visualizations help identify patterns, anomalies, and trends that may not be apparent in raw data, enabling organizations to make informed decisions and drive business growth.
AI-powered data visualization tools also provide interactive features that allow users to dive deeper into the data. Users can filter, drill down, and manipulate the visualizations to explore different scenarios and uncover insights that may have otherwise gone unnoticed. This level of interactivity empowers users to make data-driven decisions based on a comprehensive understanding of the underlying data.
Enhanced Communication and Collaboration
Data visualization with artificial intelligence not only facilitates better decision-making but also improves communication and collaboration within organizations. Visualizations have the power to simplify complex concepts and make data accessible to stakeholders across different departments and skill levels. By presenting data in a visually compelling manner, AI-powered visualizations can effectively communicate insights to teams, executives, and clients.
The interactive nature of AI-powered data visualization tools also promotes collaboration among team members. With real-time access to visualizations, team members can contribute their insights and observations, facilitating a more collaborative approach to data analysis. This collaborative environment promotes knowledge sharing and allows organizations to leverage the collective expertise of their teams for better decision-making.
Benefits of Data Visualization with Artificial Intelligence |
---|
Efficient analysis of big data sets |
Identification of patterns and trends in real-time |
Improved decision-making through data-driven insights |
Enhanced communication and collaboration within organizations |
Anomaly Detection in Big Data using AI
In the era of big data, businesses are dealing with vast amounts of data that cannot be manually analyzed. However, with the help of artificial intelligence (AI), anomaly detection has become much easier and efficient. Anomalies, also known as outliers, are data points that deviate significantly from the normal pattern or behavior in a dataset. Detecting these anomalies is crucial for businesses to identify potential issues or threats.
Challenges in Anomaly Detection
Detecting anomalies in big data comes with its own set of challenges. With the increasing volume, velocity, and variety of data, traditional methods of anomaly detection fall short. It becomes difficult to manually analyze and identify outliers in huge datasets. Additionally, anomalies can occur in diverse forms, making it hard to define a fixed rule for detection. This is where AI comes into play, offering powerful solutions to tackle these challenges.
Anomaly Detection with AI
AI techniques such as machine learning and deep learning algorithms excel in handling big data and uncovering patterns within it. These algorithms can learn from past data and use that knowledge to detect anomalies in real-time. By analyzing large volumes of data, AI can identify abnormal patterns that may go unnoticed otherwise.
There are various AI-based algorithms used for anomaly detection, including:
Algorithm | Description |
---|---|
Isolation Forest | Creates random forests to isolate anomaly instances. |
One-class Support Vector Machines | Builds a model of the normal data and detects deviations from it. |
Autoencoders | Unsupervised neural networks that reconstruct input data and detect anomalies based on reconstruction error. |
These algorithms can be applied to detect anomalies in various domains, such as cybersecurity, finance, healthcare, and manufacturing. By combining AI and big data analytics, businesses can gain valuable insights and improve decision-making processes by promptly detecting and addressing anomalies.
Real-time Big Data Processing with AI
Artificial intelligence (AI) has revolutionized the way we process and analyze big data. With the immense volume of data being generated every second, traditional methods of data processing are no longer sufficient. AI enables real-time processing of big data, allowing organizations to quickly extract insights and make informed decisions.
AI algorithms can handle vast amounts of data, identify patterns, and make predictions with a high degree of accuracy. This is particularly useful in industries such as finance, healthcare, and retail, where real-time decision-making is crucial. By using AI-powered systems, organizations can gain a competitive advantage by making faster and more precise decisions based on real-time big data analytics.
One of the key benefits of real-time big data processing with AI is the ability to detect anomalies and outliers in the data. AI algorithms can automatically identify unusual patterns or behaviors that may indicate frauds, abnormalities, or other important events. By detecting these outliers in real-time, organizations can take immediate action to mitigate risks and protect their businesses.
Another advantage of AI in real-time big data processing is the ability to automate repetitive tasks. AI systems can perform complex data analysis and generate reports automatically, eliminating the need for manual data processing. This not only improves efficiency but also reduces human error, ensuring more reliable and accurate results.
The combination of artificial intelligence and big data opens up endless possibilities for organizations to gain valuable insights and optimize their operations. With real-time big data processing, organizations can respond to changes and trends in the market faster, stay ahead of the competition, and make data-driven decisions that drive success.
Benefits of Real-time Big Data Processing with AI |
---|
1. Faster decision-making based on real-time analytics |
2. Detection of anomalies and outliers in real-time |
3. Automation of repetitive tasks for improved efficiency |
4. More reliable and accurate results with reduced human error |
5. Ability to respond to market changes and trends quickly |
6. Data-driven decision-making for competitive advantage |
Privacy and Security Challenges in AI-driven Big Data Analytics
With the increasing use of artificial intelligence in big data analytics, new challenges are emerging in terms of privacy and security. The vast amounts of data being processed and analyzed pose significant risks to individuals’ privacy if not properly protected. Additionally, the integration of AI algorithms into data analytics workflows introduces vulnerabilities that can be exploited by malicious actors.
One of the main privacy challenges in AI-driven big data analytics is data anonymization. As data is collected and stored, it is important to ensure that personally identifiable information is removed or obfuscated to prevent unauthorized access. However, this presents a trade-off between privacy and data utility, as anonymized data may lose its original value for analysis purposes.
Another challenge is the potential for re-identification of individuals through data linkage. Even if data is anonymized, it can still be possible to identify individuals by linking different datasets together. This can lead to breaches of confidentiality and privacy, as sensitive information about individuals can be revealed.
Data security is also a significant concern in AI-driven big data analytics. The integration of AI models into analytics workflows introduces new vulnerabilities that can be exploited by attackers. For example, malicious actors may manipulate the input data to an AI system in order to influence its decision-making or gain unauthorized access to sensitive information.
Privacy Challenges | Security Challenges |
---|---|
Data anonymization | Exploitation of AI vulnerabilities |
Data linkage and re-identification | Manipulation of AI input data |
To address these challenges, organizations must implement robust privacy and security measures. This includes implementing strong encryption and access controls to protect data at rest and in transit. Additionally, organizations should continuously monitor their AI systems for vulnerabilities and regularly update and patch them to mitigate the risk of exploitation.
In conclusion, the integration of artificial intelligence into big data analytics brings about new privacy and security challenges. Organizations must prioritize the protection of personal data and ensure that AI systems are secure against potential attacks. By addressing these challenges, organizations can harness the power of AI-driven big data analytics while maintaining the trust and confidence of individuals.
Ethical Considerations in the AI Revolution
The rapid growth of artificial intelligence (AI) technology has revolutionized the field of big data analytics, empowering businesses to extract valuable insights from vast amounts of data. However, with this great power comes great responsibility. Ethical considerations surrounding AI are crucial to ensuring its responsible and fair implementation.
One key ethical consideration is the potential for AI systems to perpetuate biases and discrimination. AI algorithms are trained on large datasets, which may inadvertently contain inherent biases, such as racial or gender disparities. If AI systems are used to make important decisions in areas like hiring, loan approvals, or criminal justice, these biases could be magnified and perpetuated, leading to unjust outcomes. It is essential to carefully monitor and evaluate the data used to train AI systems to minimize these biases.
Another ethical concern is the transparency of AI decision-making processes. AI algorithms often operate as “black boxes,” meaning their decision-making processes are not easily explainable or understandable. This lack of transparency raises questions about accountability and the potential for unfair or discriminatory decisions to be made without recourse. Efforts should be made to develop explainable AI models and establish regulations and standards to hold AI systems accountable for their decisions.
Privacy is another critical ethical consideration in the AI revolution. As AI systems collect and analyze vast amounts of personal data, concerns arise regarding the protection of individuals’ privacy rights. It is essential to establish secure data storage and usage practices, implement robust data protection measures, and ensure individuals have control over the use and sharing of their data.
- Integrity and trustworthiness are also crucial ethical considerations in the AI revolution. As AI systems become increasingly integrated into our daily lives, it is important to ensure their integrity and prevent malicious actors from manipulating or exploiting them for personal gain or harmful purposes. Regulations and oversight mechanisms should be put in place to ensure the ethical use of AI technology.
- Finally, ethical considerations should extend beyond the immediate impact of AI systems and encompass their long-term societal effects. It is crucial to address issues like job displacement, economic inequality, and social disruption caused by AI advancements. Ensuring a just and equitable transition to an AI-driven society requires proactive measures, including education and retraining programs, social safety nets, and policies promoting inclusive growth.
In conclusion, the AI revolution comes with ethical considerations that must be carefully addressed. Creating a responsible and fair AI-powered future requires continuous evaluation of biases, transparency in decision-making processes, privacy protection, and the establishment of integrity and trustworthiness. By considering these ethical aspects, we can harness the power of AI for the betterment of society as a whole.
Industry Applications of AI in Big Data Analytics
Artificial intelligence has revolutionized the way businesses analyze big data, providing a level of intelligence and automation that was previously unimaginable. With the power of AI, companies can now extract valuable insights and make data-driven decisions at an unprecedented scale and speed.
One of the key industry applications of AI in big data analytics is in the finance sector. Financial institutions are using AI algorithms to analyze large amounts of financial data, such as stock market trends and customer transaction records. These algorithms can detect patterns and anomalies that human analysts might miss, enabling banks to better manage risks, detect fraud, and optimize investment strategies.
Another industry that has benefited greatly from the combination of artificial intelligence and big data analytics is healthcare. AI-powered algorithms can analyze vast amounts of patient data, including medical records, imaging data, and genetic information, to help doctors make accurate diagnoses and develop personalized treatment plans. This has the potential to greatly improve patient outcomes and reduce healthcare costs.
Retail is yet another industry that is leveraging the power of AI in big data analytics. By analyzing customer purchase history, preferences, and behavior, retailers can develop targeted marketing campaigns and offer personalized recommendations to customers. This improves customer satisfaction and loyalty while optimizing sales and revenue.
Lastly, manufacturing companies are using AI in big data analytics to optimize their operational processes. By analyzing data from sensors and machines, AI algorithms can monitor and predict equipment failures, minimizing downtime and maximizing productivity. AI can also optimize supply chain management by analyzing data on inventory levels, demand patterns, and production cycles.
Industry | AI Applications |
---|---|
Finance | Risk management, fraud detection, investment optimization |
Healthcare | Diagnosis assistance, personalized treatment plans, patient outcome prediction |
Retail | Targeted marketing, personalized recommendations, sales optimization |
Manufacturing | Equipment monitoring, predictive maintenance, supply chain optimization |
These are just a few examples of how AI is being applied in big data analytics across different industries. As AI technology continues to advance, we can expect to see even more innovative applications that will transform the way businesses operate and compete in the digital age.
Healthcare and Medical Data Analysis with AI
In the healthcare industry, the amount of data generated is vast and complex. With the help of artificial intelligence (AI), healthcare organizations can now harness the power of this big data to gain valuable insights and improve patient care.
AI can analyze vast amounts of medical data, including patient records, imaging data, genomic data, and research papers. By processing and analyzing this data, AI can identify patterns, make predictions, and assist healthcare professionals in making more informed decisions.
Improved Disease Diagnosis and Treatment
One of the key areas where AI is making a significant impact is in disease diagnosis and treatment. By analyzing patient data and comparing it with vast amounts of medical literature, AI algorithms can help identify potential diseases and suggest appropriate treatment plans.
AI can also analyze medical images, such as X-rays and MRI scans, to detect abnormalities and assist radiologists in diagnosing diseases like cancer more accurately. This can lead to earlier detection and better treatment outcomes.
Predictive Analytics for Preventive Care
AI can also be used for predictive analytics to identify individuals at risk of developing certain diseases. By analyzing patient data, AI algorithms can identify patterns and early warning signs that might indicate a higher likelihood of developing a specific condition.
This information can then be used in preventive care strategies to intervene early and reduce the risk of disease progression. For example, AI algorithms can identify individuals at risk of developing diabetes and recommend lifestyle changes or interventions to prevent its onset.
- Advanced Drug Discovery and Development
- With the help of AI, drug discovery and development processes can be accelerated and made more efficient.
- AI algorithms can analyze vast amounts of genomic and molecular data to identify potential drug targets and predict the effectiveness of new drugs.
- This can help pharmaceutical companies identify promising candidates for further development and reduce the time and cost involved in bringing new drugs to market.
In conclusion, AI has the power to revolutionize healthcare and medical data analysis. By leveraging big data and the intelligence of AI algorithms, healthcare organizations can gain valuable insights, improve disease diagnosis and treatment, enable preventive care strategies, and accelerate drug discovery and development processes.
Financial and Banking Analytics with AI
The financial and banking industries generate vast amounts of data on a daily basis. With the advent of artificial intelligence (AI), these industries are now able to process and analyze this data with unprecedented speed and accuracy.
AI technology has revolutionized financial and banking analytics by providing intelligent algorithms and models that can extract valuable insights from the vast amounts of data being generated. This has enabled financial institutions to make more informed decisions and improve their overall performance.
Improved Risk Assessment
One of the key benefits of using AI in financial and banking analytics is the ability to improve risk assessment techniques. AI algorithms can analyze historical data to identify patterns and trends, allowing banks and financial institutions to better assess creditworthiness and detect potential fraudulent activities.
Through the use of machine learning algorithms, AI can also adapt and learn from new data, constantly improving its risk assessment capabilities. This enables financial institutions to stay one step ahead of emerging risks and make more accurate predictions.
Enhanced Customer Experience
AI-powered analytics can also greatly enhance the customer experience in financial and banking services. By analyzing customer data and behavior, AI algorithms can identify personalized recommendations and offers, improving cross-selling and upselling opportunities.
Additionally, AI can automate processes such as customer support and account management, reducing waiting times and providing more efficient and personalized services. This improves customer satisfaction and loyalty, leading to increased retention rates.
In conclusion, data intelligence powered by artificial intelligence is transforming the financial and banking industry. The ability to process and analyze data with advanced algorithms and models allows for improved risk assessment, enhanced customer experience, and ultimately, better performance for financial institutions.
Retail and E-commerce Analytics with AI
In recent years, the retail and e-commerce industry has seen exponential growth, with the advancement of technology playing a significant role in its success. With the increasing availability of big data, businesses are in a unique position to leverage this intelligence and gain valuable insights into consumer behavior, preferences, and trends.
The Role of Artificial Intelligence
Artificial intelligence (AI) is revolutionizing retail and e-commerce analytics by enabling businesses to process and analyze large volumes of data at an unprecedented speed and accuracy. AI algorithms can uncover patterns and correlations within the data, providing retailers with valuable information to make data-driven decisions that can lead to increased sales and customer satisfaction.
AI technologies such as machine learning and natural language processing enable retailers to analyze customer interactions, social media data, and product reviews. By analyzing customer sentiments and feedback, businesses can identify areas of improvement and enhance the customer experience. Additionally, AI can assist in dynamic pricing strategies by monitoring competitor prices, consumer demand, and market trends.
Benefits of AI in Retail and E-commerce Analytics
The integration of AI into retail and e-commerce analytics offers several benefits to businesses:
1. Enhanced Personalization:
AI algorithms can analyze customer behavior and preferences to provide personalized recommendations and marketing content, increasing customer engagement and loyalty.
2. Fraud Detection:
By analyzing patterns and anomalies in transaction data, AI can help retailers detect and prevent fraudulent activities, protecting both the business and its customers.
3. Inventory Optimization:
Through AI-powered demand forecasting, retailers can optimize their inventory levels by accurately predicting customer demand, reducing carrying costs and minimizing stockouts or overstocks.
In conclusion, the combination of big data and artificial intelligence has the potential to revolutionize the retail and e-commerce industry. By leveraging the intelligence obtained from data analytics, businesses can gain a competitive advantage, improve customer satisfaction, and enhance operational efficiency.
Manufacturing and Supply Chain Analytics with AI
With the advent of artificial intelligence (AI), the field of manufacturing and supply chain analytics has experienced a revolutionary transformation. AI has enabled companies to process and analyze big data sets with unprecedented efficiency and accuracy.
Artificial intelligence algorithms can analyze large volumes of data to identify patterns, trends, and anomalies in manufacturing and supply chain processes. This allows companies to gain valuable insights and make data-driven decisions to optimize their operations.
By leveraging AI in manufacturing and supply chain analytics, companies can improve production efficiency, reduce costs, and enhance the overall quality of their products. AI-powered analytics can help identify bottlenecks, predict maintenance needs, optimize inventory management, and streamline logistics.
Moreover, AI can also be used to identify potential risks and mitigate them proactively. By analyzing historical data and using machine learning algorithms, companies can identify potential disruptions in the supply chain, such as delays or shortages, and take proactive measures to minimize their impact.
Overall, the combination of artificial intelligence and big data analytics has revolutionized the field of manufacturing and supply chain analytics. Companies can now harness the power of AI to gain deep insights into their operations, optimize processes, and make informed decisions to drive growth and success.
Energy and Utilities Analytics with AI
As the world continues to seek sustainable energy solutions, data plays a crucial role in optimizing operations and improving efficiency. The energy and utilities sectors generate massive amounts of data, ranging from production and consumption data to weather and market information. To effectively utilize this data, big data analytics powered by artificial intelligence (AI) has emerged as a game-changer.
Application of AI in the Energy Sector
AI algorithms have the ability to analyze large datasets at high speeds, enabling energy companies to uncover valuable insights and make data-driven decisions. By leveraging AI, energy and utilities companies can optimize their operations, improve reliability, and reduce costs.
One key application of AI in the energy sector is predictive maintenance. AI models can analyze historical data, sensor readings, and other relevant factors to predict the health of critical assets such as turbines or power lines. This helps identify potential failures or performance issues proactively, allowing for timely maintenance and minimizing downtime.
AI-Driven Energy Resource Management
Another area where AI is revolutionizing the energy sector is resource management. By analyzing data from sensors, smart grids, and other sources, AI algorithms can optimize the distribution of energy resources. This includes predicting demand patterns, adjusting energy supply, and managing renewable energy sources efficiently.
Furthermore, AI can play a significant role in optimizing energy consumption. Through real-time monitoring and analysis, AI systems can identify energy waste and recommend energy-saving measures. This not only helps reduce costs for consumers but also contributes to a more sustainable energy future.
Conclusion
The combination of big data analytics and artificial intelligence has the potential to revolutionize the energy and utilities sectors. From predictive maintenance to resource management and energy optimization, AI-powered solutions can drive efficiency, reduce costs, and create a more sustainable energy ecosystem. As technology continues to advance, the benefits of AI in energy analytics will only become more pronounced, leading us towards a greener and more intelligent future.
Transportation and Logistics Analytics with AI
The transportation and logistics industry generates massive amounts of data on a daily basis. From tracking shipments to analyzing routes and optimizing supply chains, the industry relies heavily on data to make informed decisions and improve operational efficiency. However, manually analyzing this data can be a time-consuming and daunting task.
Enter artificial intelligence (AI) – a game-changer in the world of big data analytics. With AI, transportation and logistics companies can streamline their data analysis processes and gain valuable insights in a fraction of the time.
AI-powered algorithms can sift through vast volumes of data with lightning speed, identifying patterns, anomalies, and correlations that may not be immediately apparent to human analysts. This allows companies to make data-driven decisions that can drive cost savings and optimization across their operations.
One example is predictive maintenance in transportation. AI algorithms can analyze historical data, such as maintenance logs and sensor readings, to accurately predict when a vehicle or equipment is likely to fail. This allows companies to schedule maintenance proactively, minimizing downtime and reducing repair costs.
AI can also optimize transportation routes to maximize efficiency and minimize costs. By analyzing factors such as traffic patterns, customer demand, and delivery deadlines, AI algorithms can determine the most optimal routes for drivers, saving both time and fuel. This not only improves operational efficiency but also reduces carbon emissions and contributes to a more sustainable transportation industry.
Furthermore, AI can improve inventory management by analyzing historical sales data and demand patterns. By accurately forecasting demand, companies can optimize their inventory levels, reducing the risk of stockouts or overstocking. This leads to cost savings and improved customer satisfaction.
In conclusion, artificial intelligence is revolutionizing transportation and logistics analytics. By harnessing the power of AI, companies can gain actionable insights from their big data, optimize their operations, and drive cost savings. The future of the transportation and logistics industry is undoubtedly intertwined with artificial intelligence.
Telecommunications Analytics with AI
The telecommunications industry generates a massive amount of data every day. This data includes call records, network logs, customer information, and more. Analyzing this data is crucial for telecom companies to improve network performance, enhance customer experience, and identify new business opportunities.
With the advent of artificial intelligence (AI), telecom companies now have the ability to extract valuable insights from their data like never before. AI algorithms and machine learning models can analyze large volumes of data quickly and accurately, uncovering patterns, trends, and correlations that humans may miss.
Improved Network Performance
By applying AI to telecom data, companies can gain a deeper understanding of their network performance. AI algorithms can analyze historical data and identify potential network issues, such as signal degradation, congestion, or capacity limitations. This allows companies to proactively address these issues, improving network availability and reliability for their customers.
Enhanced Customer Experience
AI-powered analytics can also help telecom companies improve customer experience. By analyzing customer behavior and preferences, AI models can identify personalized recommendations and offers for individual customers. This enables telecom companies to deliver targeted marketing campaigns and enhance customer satisfaction.
Additionally, AI can be used to analyze customer feedback and sentiment data from social media and other sources. By understanding customer sentiment, telecom companies can address potential issues and improve their products and services based on customer feedback.
New Business Opportunities
AI analytics can also help telecom companies identify new business opportunities. By analyzing customer usage patterns and preferences, companies can uncover untapped markets and develop new services or products to meet customer needs. AI algorithms can also analyze market trends and competitor data to identify potential partnerships or revenue streams.
- AI-based predictive analytics can forecast demand for specific services, allowing companies to optimize their resources and plan for future growth.
- Real-time analytics powered by AI can help telecom companies detect and prevent fraud, saving millions of dollars.
In conclusion, AI has revolutionized telecommunications analytics, enabling companies to unlock the full potential of their data. With AI, telecom companies can improve network performance, enhance customer experience, and identify new business opportunities, ultimately gaining a competitive edge in the industry.
Government and Public Sector Analytics with AI
The government and public sector generate vast amounts of data on a daily basis, ranging from census data to healthcare records to transportation data. Traditionally, analyzing this big data has been a challenging task, requiring significant manpower and resources. However, with the advent of artificial intelligence (AI), these challenges are being overcome and new possibilities are being unlocked.
Intelligence is being infused into government and public sector analytics with the help of AI. Machine learning algorithms are being used to uncover patterns and insights from the immense amount of data generated by government agencies. These algorithms can identify correlations, make predictions, and provide valuable insights that can inform policy decisions and improve public services.
The Benefits of AI in Government Analytics
One major benefit of using AI in government analytics is its ability to automate processes and reduce human error. By leveraging AI technologies, government agencies can streamline their operations, increase efficiency, and save valuable time and resources. For example, AI-powered chatbots can handle citizen inquiries and provide assistance, reducing the need for manual customer support.
Another benefit is the enhanced ability to detect fraud and improve security. AI algorithms can analyze large volumes of data in real-time, flagging suspicious activities and identifying potential threats. This can help government agencies proactively prevent fraud and ensure the safety of citizens and public assets.
The Future of Government Analytics with AI
As AI continues to advance, the future of government and public sector analytics looks promising. With the integration of AI technologies, government agencies can gain deeper insights into their operations, optimize resource allocation, and make data-driven decisions that benefit society as a whole.
However, it is important to address the ethical considerations and potential biases associated with AI in government analytics. Transparency, accountability, and privacy protections should be prioritized to ensure that AI is used in a responsible and fair manner.
In conclusion, artificial intelligence is revolutionizing government and public sector analytics, enabling more efficient and effective operations, improved decision-making, and better public services.
Benefits and Challenges of Implementing AI in Big Data Analytics
Artificial intelligence (AI) has revolutionized the field of big data analytics, bringing about numerous benefits and challenges. The combination of AI technologies with big data has opened up new opportunities for businesses and organizations to extract actionable insights from vast amounts of data.
One of the key benefits of implementing AI in big data analytics is the ability to automate and streamline the data analysis process. AI algorithms can process large volumes of data at a speed and accuracy that is not achievable with traditional manual methods. This allows organizations to quickly identify patterns, trends, and correlations in their data, enabling them to make data-driven decisions more efficiently.
Another benefit of AI in big data analytics is the ability to uncover hidden insights and actionable intelligence. Machine learning algorithms can detect patterns and anomalies in the data that may go unnoticed by human analysts. By identifying these insights, organizations can gain a competitive advantage, optimize their operations, and improve their decision-making processes.
Furthermore, AI can enhance the accuracy and predictive capabilities of big data analytics. By leveraging machine learning algorithms, organizations can build predictive models that can forecast future trends and outcomes based on historical data. This enables businesses to anticipate customer behavior, identify potential risks, and make proactive strategic decisions.
However, implementing AI in big data analytics also comes with its challenges. One of the primary challenges is the need for high-quality and relevant data. AI algorithms heavily rely on data inputs for training and analysis. Therefore, organizations must ensure that their data is accurate, complete, and representative of the problem they want to solve.
Another challenge is the complexity and interpretability of AI algorithms. Deep learning and neural networks, which are commonly used in AI, can be difficult to understand and interpret. This can make it challenging for organizations to explain the decisions made by AI models and comply with regulations and ethical considerations.
In conclusion, the integration of artificial intelligence into big data analytics brings numerous benefits and challenges. By harnessing the power of AI, organizations can automate data analysis, uncover hidden insights, and improve predictive capabilities. However, they must also address the challenges of data quality and algorithm interpretability to fully realize the potential of AI in big data analytics.
Future Trends in AI-driven Big Data Analytics
In the rapidly evolving world of technology, the intersection of artificial intelligence and big data analytics has paved the way for groundbreaking advancements. As businesses strive to gather, process, and derive insights from vast amounts of data, the application of artificial intelligence in this field has proven to be invaluable.
Enhanced Data Processing with AI
With the integration of artificial intelligence, big data analytics is poised to revolutionize the way businesses extract value from their data. AI algorithms can process massive volumes of data at incredible speeds, allowing organizations to gain real-time insights and make data-driven decisions more efficiently and effectively.
The power of artificial intelligence lies in its ability to learn patterns and trends from data, enabling predictive analytics and forecasting capabilities. AI-driven big data analytics can identify correlations and anomalies that may not be apparent to human analysts, leading to more accurate predictions and improved risk management.
Moreover, AI can automate complex data processes, such as data cleansing and normalization, reducing the time and effort required for data preparation. This frees up data analysts to focus on higher-level tasks, such as data exploration and model development, resulting in more meaningful insights and discoveries.
Intelligence-infused Data Visualization
Artificial intelligence also plays a crucial role in enhancing data visualization. Traditional charts and graphs can only represent a limited amount of information, often leaving valuable insights buried deep within the data. By leveraging AI technologies, big data analytics can generate dynamic and interactive visualizations that uncover hidden patterns and relationships.
AI-driven data visualization tools can automatically identify key insights and generate visual representations that highlight the most relevant information. Machine learning algorithms can analyze the data and determine the most effective visualization techniques, ensuring that the message is conveyed clearly and concisely.
Furthermore, AI can enable natural language processing, allowing users to interact with data visualizations using voice commands or text input. This empowers decision-makers to explore complex data sets effortlessly and have meaningful conversations with their data.
In conclusion, the future of big data analytics is undoubtedly intertwined with artificial intelligence. As AI continues to advance and evolve, it will further enhance the capabilities of big data analytics, enabling businesses to make more accurate predictions, gain deeper insights, and ultimately drive innovation.
References
In the field of big data analytics, the use of artificial intelligence (AI) has revolutionized the way data is analyzed and interpreted. With AI, organizations are able to process large amounts of data quickly and efficiently, extracting valuable insights and patterns that humans may miss. AI algorithms can detect trends, predict future outcomes, and identify anomalies in the data.
One of the key advantages of using AI in big data analytics is the ability to automate repetitive tasks. This allows analysts to focus on more complex and higher-value tasks, such as developing new data models or creating innovative data visualizations. AI algorithms can also learn from patterns in the data, improving their accuracy and effectiveness over time.
AI-powered big data analytics tools are being used in a wide range of industries, from finance and healthcare to retail and manufacturing. These tools are helping organizations make more informed decisions, optimize their operations, and gain a competitive edge.
As the field of AI continues to advance, so too will its impact on big data analytics. New AI techniques and technologies, such as machine learning and natural language processing, are constantly being developed and refined. This will enable even more sophisticated analysis of big data, leading to enhanced insights and outcomes.
In conclusion, the combination of artificial intelligence and big data analytics is transforming the way organizations process and analyze data. The use of AI algorithms allows for faster and more accurate analysis, resulting in actionable insights that can drive business success.
Questions and answers
How is artificial intelligence revolutionizing big data analytics?
Artificial intelligence is revolutionizing big data analytics by automating the process of analyzing and interpreting large amounts of data. AI algorithms can extract valuable insights from data much faster and more accurately than humans, enabling businesses to make data-driven decisions with confidence.
What are some examples of how AI is being used in big data analytics?
There are several examples of AI being used in big data analytics. One example is predictive analytics, where AI algorithms can analyze large datasets to identify patterns and make predictions about future outcomes. Another example is natural language processing, where AI can process and analyze unstructured data, such as text or speech, to extract meaningful information.
How can AI help businesses make better use of their big data?
AI can help businesses make better use of their big data by automating the analysis process and extracting valuable insights. With AI algorithms, businesses can quickly identify trends, patterns, and correlations in their data, allowing them to make data-driven decisions and gain a competitive edge.
What are the benefits of using AI in big data analytics?
There are several benefits of using AI in big data analytics. First, AI can process and analyze large amounts of data much faster than humans, saving time and resources. Second, AI algorithms can identify patterns and trends that may not be apparent to humans, leading to more accurate predictions and insights. Finally, AI can automate repetitive tasks, allowing data analysts to focus on higher-level tasks that require human expertise.
What are some challenges of implementing AI in big data analytics?
There are several challenges of implementing AI in big data analytics. One challenge is ensuring the quality and accuracy of the data used for training AI algorithms. Another challenge is the complexity of AI algorithms, which may require specialized skills and expertise to develop and maintain. Additionally, the ethical implications of using AI in big data analytics, such as privacy concerns, need to be carefully considered and addressed.
How does artificial intelligence revolutionize big data analytics?
Artificial intelligence revolutionizes big data analytics by allowing computers to analyze and interpret massive amounts of data much faster and more accurately than humans. AI algorithms can identify patterns, make predictions, and provide valuable insights from complex data sets. This leads to more efficient decision-making, improved business strategies, and enhanced productivity.
What are some examples of how artificial intelligence is used in big data analytics?
There are several examples of how artificial intelligence is used in big data analytics. One example is the use of machine learning algorithms to train models that can predict customer behavior, preferences, and outcomes. Another example is the use of natural language processing, which allows computers to understand and analyze unstructured data such as social media posts or customer reviews. AI algorithms can also be used to automate data collection, cleaning, and analysis processes, saving time and resources.