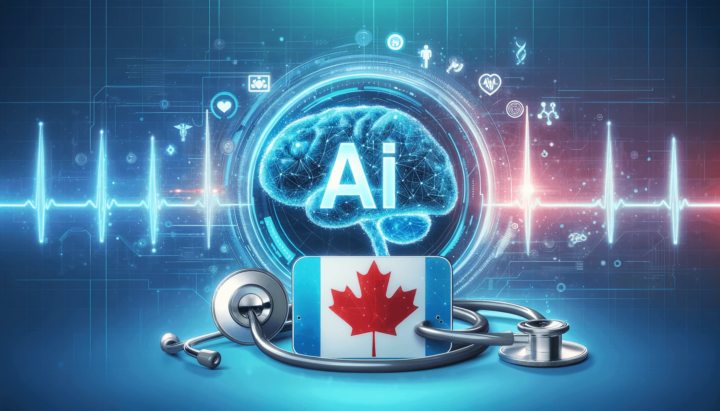
Introduction to AI in Healthcare
Definition of AI in healthcare
Artificial Intelligence (AI) refers to advanced computer systems and software that can analyze data, learn from it, and make decisions or recommendations modeled after human intelligence and predictive capabilities. In a healthcare context, AI technologies are being applied across the care continuum to derive data-driven insights, enhance decision-making, automate administrative tasks, and improve patient experience.
Key techniques powering AI in healthcare include machine learning, natural language processing, computer vision, predictive analytics and robotics – leveraging the vast troves of multimodal patient data to unlock personalized and more effective treatments. As AI capabilities continue maturing, it is poised to transform how care is delivered.
Historical development of AI in healthcare
The concept of artificial intelligence has been around since the 1950s, but only recent expansions in computing performance combined with exponential growth of health data has made practical applications in medicine possible.
Some key milestones in AI in healthcare include:
- 1950s – First neural network algorithm based on human brain created
- 1960s – Early expert systems for medical diagnosis emerged
- 1980s – Machine learning techniques gained traction for clinical decision support
- 1990s – Natural language processing entered for medical records analysis
- 2000s – AI adopted for genomic data mining and drug discovery
- 2010s – Deep learning breakthroughs enable precision diagnostics
- 2020s – COVID-19 accelerated AI adoption for telemedicine, hospital operations and vaccine development
As algorithms continues advancing in tandem with health data aggregations, the roles for AI in transforming care continues expanding.
Current landscape of AI applications in Canadian healthcare
Canada has emerged as a global leader in AI research and adoption thanks to world-class data science capabilities combined with provincial health organizations prioritizing next-generation technologies. An expanding roster of health-focused AI startups have taken root leveraging the country’s digitized data assets.
Some current areas witnessing AI innovation across Canadian healthcare encompasses:
Clinical Practice
- Diagnostic support – e.g. algorithms analyzing medical images for abnormalities or robotic surgery platforms
- Patient triage and symptom assessment via apps
- Optimizing medication prescriptions and dosing adjustments leveraging pharmacogenomic data
- Predictive analytics to anticipate risks of hospital readmissions helping preventative care
Public Health
- Early warning systems by finding signals in non-traditional data sources indicating disease outbreaks
- AI simulation models guiding priorityplanning around vaccines or resource allocation amid contagions
- Personalized digital coaching nudging lifestyle changes lowering population disease predisposition
Administrative
- Automating paperwork allowing clinicians more time with patients
- Improving scheduling and coordination of care delivery across staff functions via process mining
- AI cybersecurity assistants safeguarding systems against threats
As health data volumes keep growing, computers keep getting faster and algorithms smarter – the applications are rapidly expanding.
Future prospects and challenges
According to recent surveys, close to 80 percent of Canadian healthcare delivery organizations are actively implementing AI solutions indicating the immense perceived potential.
As machines get better at consuming more data contextually,redundant tasks can be automated freeing up human focus for complex case management and relationship building underscoring healthcare’s service ethic. Already algorithms match specialists in accuracy for certain pathology detections from scans or fitness trackers enable round-the-clock monitoring.
However distrust in blackbox recommendations can undermine adoption. That necessitates new explainable methodologies building confidence in AI judgement. Technologies failures also requires updated legal frameworks apportioning culpability. So the journey promises exciting but requiring thoughtful governance addressing emerging risks like data discrimination, therapeutic automation lacking empathy and consent management upholding dignity.
Getting AI design and evaluation right can catalyze gains across diagnosis speeds, error reductions, procedural efficiency and patient experiences realizing productivity aspirations without compromising ethics. So progress must uphold both better health outcomes and humanistic priorities fundamental to caregiving or risk backlash stymieing support. Still it remains a promising avenue if guided responsibly.
Regulatory Framework and Standards
Health Canada’s regulations for AI in healthcare
Health Canada plays an important regulatory role shaping healthcare technology adoption to balance innovation support and safety assurances. For AI based tools, recently proposed guidance differentiate various risks classes directing customized oversight like:
Minimal risk – e.g. algorithms improving workflow efficiencies – necessitate internal quality checks before allowing modifications and spread. Low risk – e.g. drug dosing assistants – require external validation testing ensuring recommendations align with standard protocols without significant deviations. Higher risk – e.g. diagnostic pattern recognizers – necessitates pre-market third party algorithmic auditing and post-deployment monitoring ensuring sustainability.
Labels proportionate to performance claims also foster appropriate reliance and accountability. Overall the regime focuses catalyzing innovation in responsible ways upholding patient rights.
International standards and best practices for health datasets used in AI-based applications
Since dataset biases can propagate blindspots limiting model reliability necessitating governance standards around curation, like:
- Representativeness – Ensuring inclusive population coverage not skewed by easier digitization of majority demographics
- Labelling integrity – Precise classification niether overlooking risk factors nor falsely escalating ordinary variations
- Interoperability – Normalizing metadata formats, lexicon of terms etc facilitating consolidation across sources
- Provenance – Metadata on origin, prior applications enabling impact audit trails
- Deidentification – Anonymizing individual identifiers balancing analytical utility and personal privacy
Such protocols help enhance data quality available for algorithm training uplifting reproducibility, auditability and fairness.
Safety regulations for health-related AI
While the benefits potential for AI healthcare tools creates understandable enthusiasm, patient wellbeing necessitates assurance safeguards given software complexities. Core elements involve:
Risk-adaptive expectations – Based on intended uses, tailored guidelines indicates required external validator credentials, evaluative rigor thresholds, evidence reporting protocols etc. mapped proportionately to harms possibilities
Transparency mandates – Revealing underlying training data characteristics, modeling techniques, theoretical causal explanations etc. builds confidence allowing stakeholders assessing strengths-weaknesses
Uncertainty tracking – Measurement statistics quantifying precision, estimated error rates for various scenarios helps contextualize interpretations flagging low reliability outputs warranting confirmation
Life cycle governance – Monitoring mechanisms tracking key indicators across software versions maintaining model robustness and tool usefulness amid practice shifts
Such comprehensive protocol creates quality standardization accelerating adoption.
Software and AI as a Medical Device Change Programme in Canada
As advanced analytics functionalities applied on health data can guide clinical determinations, questions surface on appropriate oversight protocols. Health Canada recently constituted the Digital Health Review Division to optimize regulatory pathways maintaining safety and innovation.
Some key aspects for software and AI based tools include:
Risk-tiered expectations – Based on intended functionality, tailored guidelines outline verification & validation needs before allowing marketing
Real world performance measures – Post-launch learning health frameworks continually gather statistics detecting model deterioration signals prompting retraining as environments evolveExplainability principles – Ensuring model behaviors links clearly to underlying causal factors vs spurious correlations uplifting reliability and trustEthics review mandates – External boards providing non-binding recommendations help align profit motivations with patient priorities steering responsible innovation
By recognizing unique facets necessitating updated thinking for computational medicine, customized modern governance helps catalyze access advancing health equity.
Clinical Applications of AI
Diagnostic applications of AI in Canadian healthcare
AI is demonstrating immense potential improving and expediting disease diagnoses – a critical determinant of positive outcomes. For instance, alongside obvious technical advantages in speed and data processing scale, algorithms match or even outperform specialists in analyzing medical images for critical conditions often with greater reliability while working 24/7 lowering costs.
Enhanced digital acquisition generating more diagnostic data combined with more powerful AI means their roles will greatly expand in years ahead. Already Health Canada is expanding approvals for marketing such tools applied in areas from spotting diabetic retinopathy indicators to classification of breast cancer malignancies or rheumatological condition activity enabling earlier actions.
Therapeutic applications of AI in Canadian healthcare
Beyond diagnostics, AI is also optimizing interventions from clarifying best medication choices by modeling predicted biochemical interactions and effects considering genomic variabilities to dynamically personalizing radiation therapies adapting to morphological changes minimizing collateral organ damage.
Canadian researchers have demonstrated AI efficacy matching multi-disciplinary tumor boards in identifying ideal immunotherapy combinations or disease stage-specific dosage guidance. Such evidence-anchored recommendations helps clinicians maximize outcomes.
As predictive simulations get more granularly accurate and powerful, expanding therapeutic automation can uplift productivity and efficacy but requires updating medical ethics education on appropriate human judgement positioning.
AI in medical imaging and radiology
Medical imaging combined with AI represents a major segment driving more precise diagnoses and transforming patient pathways. Algorithms can be trained on millions of labelled scans picking up patterns human clinicians might miss revealing subtle but significant signs enabling earlier confident discovery when treatment chances remain more favorable.
For instance MetaOptima, a spinoff from a Vancouver hospital, developed an AI system to review optical coherence tomography (OCT) scans for signs of chronic diabetes related eye damage due to high blood sugar. Diagnosing such issues early is key to prevent vision loss via interventions. Their algorithm matches world-leading experts and has received Health Canada licensing.
As more such assistants emerge targeting detection of various conditions from brain clots and tumors or types of lung disease, radiologists are freed to focus on harder cases. Pre-analyzing scans speeds overall workflows allowing faster conveyance of results to specialists for confirmation accelerating care access and lifesaving treatment.
AI in drug discovery and development
The exhaustive process from initial compound discoveries to optimized molecule candidates for illness treatment pathways can take years necessitating massive investments, but AI is drastically shrinking this using simulation models.
For starters, AI methods can swiftly screen millions of molecule specification combinations judging affinity to bind with high accuracy to intended disease targets indicating promise. Thereafter predicting downstream impacts relies on understanding complexity from protein folding physics to biochemical interactions. Modern computational power trains complex neural nets capable of unpacking such intricacy.
Startup Cyclica leverages such capacity to design innovative treatments. Recently they created a coronavirus protease inhibitor for COVID therapy in just few months versus years conventionally. With further advances, costs can lower as investments get derisked via more successes and faster patient impact.
Ethical and Legal Considerations
Ethical guidelines for AI in healthcare
As AI permeates healthcare, thought leaders have focused on building an ethical framework guiding developments focused on moral ends. Core principles include:
Beneficence – Ensuring patient wellbeing remains the north star prioritizing benefits over profits
Non-maleficence – Rigorously testing for unintended harms to safeguard against avoidable deterioration
Autonomy – Granting self-determination rights via informed consent and authority to override technology guidance
Justice – Committing applications first targeting marginalized groups advancing equity before majorities
Operationalizing such aspirations relies on cross-disciplinary teams providing multifaceted inputs shaping innovation priorities through participatory processes converging to humanistic technology.
Legal implications of AI in Canadian healthcare
Emerging AI capabilities pose tricky challenges for Canadian healthcare’s legal contours as lines blur on aspects like authority primacy and culpability. If machines triaging patients err or customized therapy protocols cause complications, disputes arise determining negligence burdens across technical creators, hospital adopters and oversight physicians.
Precedent setting case laws are still evolving which will influence innovation pathways balancing responsibility and advancement. In the interim clearly specifying software limitations sets reasonable expectations allowing proportional liabilities if harms emerge. Ultimately the law must uphold access and ethical practice accommodating context sensitive AI benefits while clarifying residual roles for unquantifiable human judgement sustaining patient trust.
Patient privacy and data security in AI applications
Centralizing large volumes of patient data inevitably heightens privacy risks necessitating rigorous access controls and anonymization. PIPEDA and provincial laws safeguards healthcare info directing technical protections like multi-factor authentication, compartmentalized data stores and metadata auditing to trace breaches.
Deidentification via coding healthcare data before computation maintains anonymity. Privacy certification regimes further upholds sensitivity mandating periodic third party auditing attesting compliance. Such comprehensive measures strives upholding confidentiality amid essential health analytics.
Informed consent and transparency in AI-based healthcare interventions
Seeking patient buy-in for diagnostic or treatment pathways guided by AI assisted recommendations requires clarity on data inputs, logics driving interpretations and possible probabilistic outcomes respecting ethical disclosure duties. Customizable consent procedures catering to literacy compatibilities coupled with options to override technology suggestions or speak to human clinicians upholds autonomy.
Responsible innovation also necessitates explainable AI models allowing physicians understanding logic links between presented information and conclusions verifying reliability so they remain ultimately accountable for patient care quality. Together such transparency nurtures collaborative clinician-computer partnerships enhancing both productivity and trust.
Health Equity and Representation
Addressing bias and fairness in AI algorithms
Like any technology, AI risks entrenching structural biases and inequities if deployed without deliberate quality controls. Thoughtfully addressing aspects like:
Representative data coverage – Ensuring marginalized community inclusion training datasets, not just majority demographicsRigorous caveat testing – Proactively probing performance on outlier scenarios unearthing generalization gapsCommunity based auditing – Gathering external feedback beyond technical metrics steering priorities centered on lived experiencesGuardrail algorithms – Secondary neural nets checking primary model outputs for prejudices flagging questionable recommendations
Such steps improves model responsiveness to all patients needs upholding dignity.
Ensuring health equity in AI-based healthcare
Thoughtful AI implementation presents opportunities uplifting communities historically underserved by conventional care models via tools improving awareness, access and affordability. For instance chatbots bridging geographic barriers or computer vision expanding diagnostics reach remote towns balanced by risks of worsening disparities if deployment focuses affluent segments first.
But prioritizing marginalized groups user needs through participatory design processes combined with metrics tracking population coverage sustains equity. Fundamentally solutions must remain anchored around patient priorities rather than technological possibilities alone if AI aims to distribute quality care fairly by overcoming past imbalances.
Representation of diverse populations in AI datasets
Training datasets form the foundation uplifting algorithm robustness. But typically digitization begins with majority demographics limiting model reliability for minority groups. Broadening input data coverage to represent all populations ensures learnings encapsulate a spectrum of genetics, lifestyles and environmental factors.
But historical barriers around outreach and trust makes gathering representative data difficult though essential. Improved engagement strategies centered on mutual benefit and investigational partnerships provides paths forward upholding diversity sufficiently training AI that benefits communities comprehensively.
Coding and categorization of minoritized and marginalized groups
Classifying patient groups when labeling AI training data risks misrepresentation if relying purely on technical categorizations rather than honoring self-identified attributes respecting lived identities. Ethically gathering community preferences guides taxonomy structures accurately encapsulating granular distinctions.
Then codifying alignment with administrative definitions e.g. for ethnicity enables standardized population scale analytics while upholding personal dignity. Consultative processes balancing technical utility and social humility sustains trust enabling inclusive AI progress.
Integration of AI into Canadian Health System
Challenges and opportunities of integrating AI in Canadian healthcare
Optimizing AI integration hinges on aligning tool capabilities with clinical workflows solving pressing pain points while addressing adoption friction factors like disruptive system changes or technologist-clinician goal mismatches which undermine reliability and utilization. This requires insights mapping institutional decision making balancing both human and technical capability advances thoughtfully.
Role of electronic health records in AI adoption
Vast troves of patient data scattered across electronic medical records, insurance claims systems and genetic testing repositories remains trapped in inconsistent formats and incomplete context challenging analytics. Modern natural language processing and ontological mapping methods can extract and organize such data into analyzable data lakes better revealing longitudinal insights for algorithmic diagnostics and predictive triage.
Updated data interoperability frame-works removing silos while maintaining privacy enables scaling such efforts unlocking healthcare treasure troves benefiting research and practice.
Collaboration between healthcare institutions for AI implementation
Fragmented development creates algorithmic solutions ill-fitting for frontline practices wasting investments. But meaningfully convening health systems, academia and industry via participatory processes grounds implementations to user realities.
Joint oversight further allows navigating regulatory uncertainties, mitigating community risks and scaling capacity investments benefiting all leveraging AI equitably. Ultimately realizing returns necessitates breaking down barriers across sectors bringing strengths together.
Impact of AI on healthcare workforce and patient care
Emerging AI capabilities handling structured tasks like diagnostics evaluation or documentation review threatens certain job categories. However the productivity efficiency gains also alleviates physician shortages granting more availability for patient engagements, complex case management and leadership oversight realigning roles to human strengths while embracing data-driven augmentation.
Still transition support remains vital helping clinical experts best orient priorities balancing AI partnerships. Getting this balance right promises enhancing both economic and humane ends.
Data Governance and Privacy
Data governance frameworks for AI in Canadian healthcare
Centralizing vast patient info volume elevates privacy risks necessitating governance upholding security and trust. Core elements involves:
Custodial responsibilities – Clear roles across data capture, storage, applications outlining accountabilities and access protocols institutionalizing checks avoiding misuse
Provenance documentation – Metadata recording source, prior usage, transformation logics etc enabling impact audit trails determining model reliability
Limited durations – Mandating data deletions post-project lifecycle minimizes exposure footprint
Algorithmic explainability – Model interpretability methodologies clarifying computational logics builds stakeholder trust and refinement opportunities
Such clarity upholds ethical obligations amid essential health analysis.
Privacy regulations and data protection laws
Healthcare’s sensitivity means managing appropriate data usage relies on minimally intrusive collection needs, robust protections against unauthorized access and accountability mechanisms tracing breaches. In Canada, PIPEDA outlines expectations on transparent consent processes while public sector data laws detail technical control requirements meeting enterprise-grade standards directing design obligations.
Data sharing and interoperability in AI applications
Data trapped in siloed systems drastically limits AI training set diversity undermining reliability. Updated architectures outlining standardscompliant interchange formats, controlled access mechanisms and decentralized analytics expands availability upholding privacy enabling robust modelling in turn unlocking productivity and equity.
Oversight authorities play a key role convening hospitals to develop aligned data sharing policies navigating competitive tensions. Expanding data trusts also provide legal models facilitating appropriate sharing targeting the greater good.
Ethical use of patient data in AI algorithms
Expanding reliance on patient data for developing AI models raises ethical questions around reciprocity and inadvertent harms. Deliberately addressing aspects like community protections, proactive risk sharing allowing participatory shaping and prioritizing marginalized group members needs before profitable segments sustains ethical data sharing upholding public trust while still advancing innovation for societal benefit.
Ultimately AI should uplift everyone in keeping with healthcare’s service ethic instead of disproportionately benefiting particular groups. This demands data governance frameworks centered on moral philosophy rather than just property laws alone.
AI and Medical Device Regulations
Health Canada’s approach to AI as a medical device
AI’s data processing scale producing diagnostic and treatment suggestions necessitates updated device regulations balancing safety assurances and innovation support. To enable real-world learning and adaptation, Health Canada’s Digital Health Review Division constituted staged approaches with controls proportionate to risk profiles linked to intended uses. This allows incremental approvals fine tuning larger implications before unrestricted authorization.
Pre-and post-market evidence gathering further bolsters detection sensitivities around safety and effectiveness upholding standards. Taken together it promises responsive oversight keeping patients first while enabling modern AI benefits.
Licensing and approval process for AI technologies
Determining appropriate oversight mechanisms matching AI techniques with risk profiles remains complicated by rapid evolvement. So Health Canada delineates adaptive guidelines classifying functionality nuances, building interpretability expectations and stipulating human factor needs allowing staged introduction respecting both patient wellbeing and diagnostic potential.
Tailored accreditation regimes also promote technology developers committing to transparency and post-market vigilance through proactive monitoring systems upholding stakeholder trust as AI assistants permeates healthcare.
Digital Health Review Division within Health Canada
The novel considerations needing governance around digital health tools has led Health Canada to constitute a targeted division optimizing oversight keeping pace with software-based innovation. Core focuses include:
Customized review pathways – Staged approvals allow incremental feature introductions updated responding to learningsRisk-tiered acceleration – Prioritizing unmet need solutions e.g. rare disease diagnostics advances equityPatient safety standards – Model validation prerequisites proportionate to performance claims maintains ethicsCybersecurity – Mandating controls against unauthorized data access or functionality creeping
Together this promises optimizing access and advancement upholding checks and balances benefitting all.
Reforms and challenges in AI as a medical device
Updating traditional quality assurance thinking for adaptive AI/ML technologies but still upholding scientific vigor around aspects like clinical validation necessitates regulatory reforms striking balances.
Emerging complication areas include:Real world evidence – Retraining on newly misclassified examples risks instilling biases limiting generalizabilityAlgorithmic opacity – Impenetrable model logics challenges explainability measures upholding understandingValidation accretion – Gradual performance improvements means moving compliance goalpostsLegacy technique alignment – Raising oversight standards risks limiting conventional technique availability
Still, progress centering ethical ends remains imperative guiding innovation trajectories responsibly.
AI in Low and Middle Income Economies
Disparities in AI development for healthcare between high-income and low-middle income countries
A widening chasm exists around AI availability for health needs between industrialized and emerging contexts. Estimates indicate over 90 percent of healthcare AI focused startups originate higher-income nations but could disproportionately benefit global south addressing gaps.
However data limitations, technical skills shortages, healthcare accessibility divides and affordability barriers curtails development partnerships undermining collective potential. Still concerted efforts centered on cooperation can proliferate capabilities more equitably.
Collaborative efforts for AI in healthcare in LMICs
While equitable development challenges limit immediate standalone opportunities, emphasizing mutually beneficial arrangements and public-private development partnerships around technology assistance, contextual knowledge exchange and joint oversight boards upholding aligned interests provides paths forward.
Platform models allowing modular analytics components also de-risk smaller incremental investments scaling impact. Such creative bridge building offers expanding gains more inclusively upholding healthcare universally.
Impact of AI on Universal Health Coverage and Sustainable Development Goals
Thoughtfully governed AI adoption holds possibilities transforming healthcare accessibility upholding universal coverage and sustainable development goals by:
- Optimizing limited specialist reach via tele-medicine
- Informing resource planning and priority allocation using predictive analytics
- Uncovering service gaps analyzing records informing system reforms
- Improving patient triage and population risk stratification targeting prevention
Still enabling progress relies on global partnerships uplifting marginalized community data value chains first where needs remains greatest. Ultimately data and decisions must aid the underserved worldwide.
Building a collaborative ecosystem for AI in healthcare in LMICs
A shared mission focused on expanding quality healthcare access by responsibly leveraging AI’s potential can catalyze progress transcending borders. Elements for such an ecosystem include:
Innovation hubs providing technical support lowering barriers translating research to implementationsCo-designed interventions built grounds up aligning clinical realities and algoritmic capabilitiesData trusts enabling access to external datasets while upholding regional privacyCloud support overcoming storage and security limits expanding analytics capacity
Together this promises uplifting communities currently underserved by AI globally democratizing gains more equitably.
Future of AI in Canadian Healthcare
Predictive analytics and personalized medicine
Already AI assistance is standardizing previously imprecise diagnostics matching specialists while symptom checkers and chatbots triages early risks. Soon virtual health twins analyzing individual health histories may foresee illness trajectories years before onset allowing preemptive therapies averting disease progression.
Pharmacogenomic medication recommendations today promises extending into full genome guided interventions predicting optimal protocols maximizing positive outcomes minimizing adverse reactions based on a patient’s unique biology. Much risk and costs promises mitigation accelerating access and advancement.
AI in public health and disease surveillance
AI simulation models today spots potential viral mutation threats and models epidemic trajectories guiding containment policies. Soon analyzing environmental toxin spread patterns may uncover previously unidentified predisposition sources supporting regulations minimizing population harm.
Meanwhile predictive analytics pinpointing communities exhibiting emerging outbreak indicators can mobilize proactive interventions containing spread nimbly upholding health system integrity and community wellbeing simultaneously.
Innovations in AI-enabled healthcare delivery
Already telemedicine platforms offers on-demand access to physicians while home based sensors monitors patient vitals minimizing hospital visits for chronic disease care. Soon low cost augmented reality glasses may overlay patient scans with anomalies highlighted that novices can interpret democratizing global diagnostic reach.
Meanwhile adverse event detectors continually assess electronic records can alert risks predicting complications helping prevention. Together a revolution promising far more personalized, preventative and participatory care emerges powered by AI proliferation.
Investment and funding trends in AI for Canadian healthcare
Already Canadian healthcare AI startups attracted record $740 million venture funding in 2023 indicating massive perceived potential supporting tools from machine learning powered patient triage applications to AI-guided robotic surgery analytics.
Major hospital networks are also launching inhouse AI accelerator programs tapping local data assets further attracting global partnerships promising extending care access powered by exponentially improving predictions unlocking both productivity and quality gains benefitting all of society.
AI and Public Health Preparedness
Role of AI in public health preparedness
Mobilizing coordinated pandemic responses relies on real-time visibility into disease indicators and population coverage metrics informing policy planning containing spread balancing health and economic impacts.
AI predictive models forecasting likely infection trajectories based on mobility patterns and genomic virus characteristics allows proactive capacity building targeting probable hotspots minimizing community disruption through data driven social distancing priors. Modern analytics promises bringing needed speed and precision improving readiness saving lives.
AI-enabled initiatives for improving service delivery and health outcomes
Challenges managing screening intake volume for preventable diseases like diabetes or cancer risks delayed identification allowing complications. Machine learning based personalized risk calculators analyzing longitudinal records stratifies patients on progression likelihood guiding scheduling prioritizing higher probability cases first while managing routine intervals for lower risks optimizing capacity and staging treatment aggressiveness proportionately.
Integration of AI innovations with existing health programs
Scaling promising AI toolkit relies on seamless embedment into clinical workflows maintaining coordination continuity. For instance natural language chatbots gathering patient reported symptoms ahead appointments triages allowing physician directed diagnostic escalations or deferrals freeing up in-person time for physical assessments uplifting overall care capacity through appropriate delegation between human and machine capability.
Collecting representative health data for AI health interventions
Training reliable AI necessitates quality datasets but historical access barriers makes gathering representative data across populations challenging limiting intervention generalizability. So inclusive consultation designing ethical outreach incentives centered on mutual benefits combined with aggregated anonymization upholding personal privacy provides paths improving AI effectiveness for all communities.
AI and Universal Health Coverage
AI’s role in achieving Universal Health Coverage
Thoughtfully governing AI adoption holds possibilities transforming healthcare accessibility – optimizing limited specialist reach via tele-medicine and remote diagnostics, informing resource planning and priority allocation using predictive analytics, uncovering service gaps analyzing patient records and experiences while improving population risk stratification targeting prevention interventions to at-risk groups.
Such capabilities can unlock efficiency gains extending coverage for marginalized communities advancing universal health through data driven insights balancing system limitations.
Impact of AI on health system preparedness and resources
As climate change escalates contagion risks, early outbreak interception helps containment protecting capacity integrity. Analyzing population media consumption patterns using natural language processing for queries on symptoms in typically mundane flu enables signal differentiation and location-based mobilization visualization dashboards guiding targeted responses specific to neighborhood needs.
Global commitment on digital health and AI
Equitable access to quality healthcare services relies on responsibly accelerating technology adoption solving entrenched delivery gaps. Committing sustained investments into participatory AI partnerships elevating tools addressing accessibility, affordability and accountability barriers with priority for marginalized groups upholds driving universal coverages through smart design processes guided morally.
Minimizing gaps in health system preparedness through AI initiatives
Resource starved contexts battles balancing priorities spread thinly but data driven insights promises informing threat anticipation and outbreak likelihoods using AI modelling techniques on lead indicators from digital disease detection tools. Guideposts assessing differential preparedness gaps allows targeting interventions averting crisis scenarios upholding system integrity continuity serving communities protecting universal wellbeing.
AI is rapidly advancing Canadian healthcare unlocking productivity and quality gains if thoughtfully and ethically governed addressing aspects of transparency, accountability, sustainability, privacy and accessibility centering patient dignity.
Technological capabilities now increasingly match clinician capacities across diagnostic accuracies to data volume comprehensions – extending reach and repeatability of top expertise benefiting more people predictively and preemptively while still necessitating human partnerships upholding emotional bonds fundamental to caregiving transcending any quantification.
Therefore future progress interweaving human and machine intelligence symbiotically promises enhancing access, outcomes and experiences while upholding essential humane relationship so core to healing. But this requires updated skills mapping strengths appropriately, participatory design processes uplifting underserved voices first and refreshed oversight balancing innovation aspirations and moral protections sustaining healthcare’s virtues while unlocking new possibilities benefitting all.
Ultimately responsible shepherding focused on care and community can catalyze new data-enabled clinical capabilities making equitable quality healthcare accessible to everyone in the country and around the world.